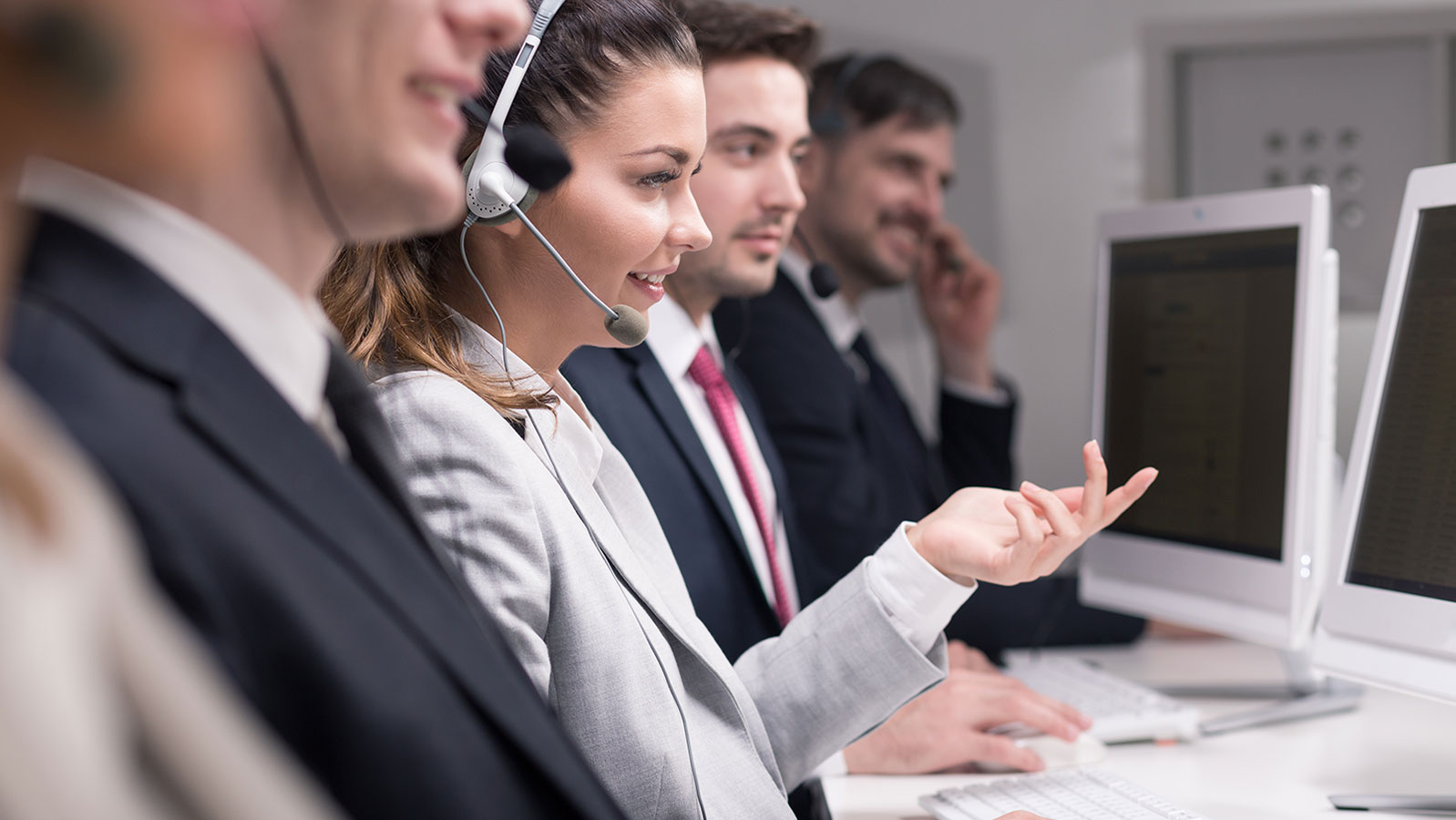
How AI Help Banks Plan for a Surge in Non-Performing Loans
We’re in the midst of an economic crisis unlike anything we’ve faced before. Driven by a year-long pandemic and sudden halt of economic activity due to the coronavirus outbreak, the economy has shed trillions of dollars in wealth, and tens of millions of Americans are now out of work.
That pain is being felt across the economy – from restaurant workers suddenly out of work to banks with significant holdings in volatile loan markets. In particular, the commercial mortgage market is at a high level of risk. Colony Capital Chairman Tom Barrack described it as “on the brink of collapse” and that without immediate action to address loan restructuring to weather the current crisis, there could be a catastrophic failure across the market.
The massive volume of debt held by the world’s banks is at significant risk, not just due to the severity of the current economic crisis but also due to the speed with which it is accumulating. The 2009 financial crisis that took down one of the world’s largest financial institutions and forced dozens more to seek bailouts from world governments took months to formulate. The March 2020 stock market crash took just a couple of weeks. According to Goldman Sachs, the current state of non-performing loans, regardless of which part of the world you live in, is at a staggering level. Artificial intelligence is bound to play a major role in protecting against and responding to this unprecedented crisis. AI can enable banks to implement revenue recovery/restructuring efforts that can, in turn, achieve better outcomes with increased revenue, better call success ratio, and increased number of satisfied customers due to a higher first call resolution rate. Let’s take a closer look at how AI integrates into these solutions and what can be done to better leverage these opportunities.
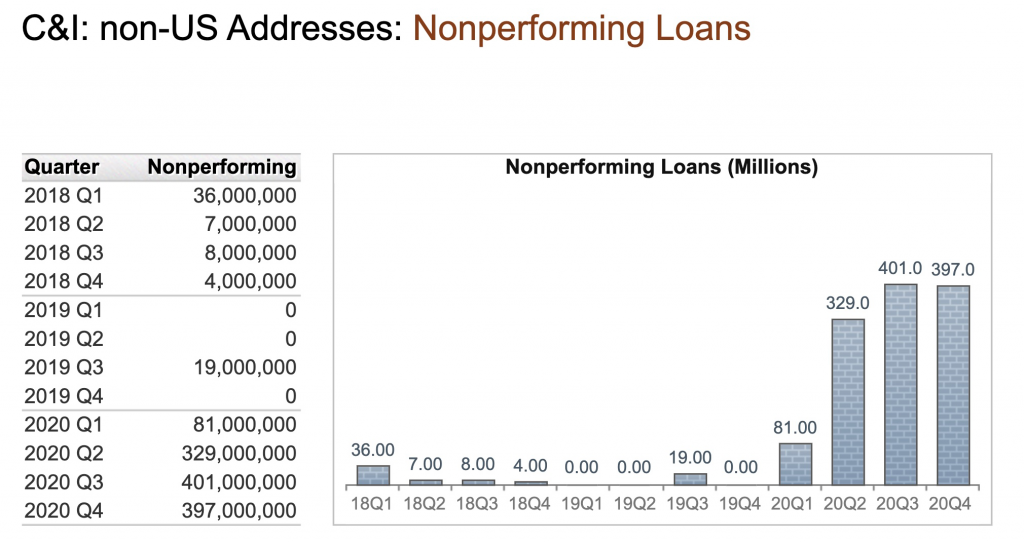
The Role of AI in Preparing for Financial Havoc
Banks already heavily rely on AI to supplement their analysis and response to major events. While rare, black swan events like the 2009 financial crisis and the coronavirus outbreak can be catastrophic without a plan in place to respond to it quickly. AI is, therefore, being used to evaluate historical data and model likely outcomes in the event of such an occurrence. In part, this is why the stock market sank so much so quickly before stabilizing as computer trading algorithms quickly priced in the likely impact of business closures and illness.
To effectively prepare for these kinds of events, financial institutions are recommended to build a model of their business that can respond to simulations of potential issues. While we are currently in the midst of just such an event, it can still be helpful to evaluate the likely outcomes of the recovery effort over the next 12-18 months. What that process looks like, and the potential responses we’ll see, both in the economy and from the government will impact the economy.
Ernst & Young released a report stating that AI will play a role beyond simply supporting and amplifying current financial products. It will become the core of a personal financial operating system that allows individualized flexibility for financial products without added effort from banks. AI has the power to break banks out of a product-based business model that packages and repackages the same old loans and financial products in a new way using technology. By gathering data and utilizing it to inform not just products offered, but the means by which an organization operates, banks can transform their service offerings, even in the midst of a crisis to respond in real-time to unexpected events.
The Role of AI in Addressing Non-performing Loans
The number of non-performing loans (NPLs) during the 2009 Financial Crisis doubled from pre-crisis levels. The COVID19 pandemic has thrown these levels into staggering heights. By the end of 2020, the US had $127.6bn (up from $95bn end of 2019) in NPLs, and Europe was battling a €401bn wave of new in-the-red loans. Entering 2021 this will represent a significant strain on the banks. At the same time, the current methods of revenue recovery in contact centers are inefficient and largely driven by human operators and analysis. AI-Mediated Conversations and predictive models that evaluate customers in real-time can help to transform this process with better profiling and prioritization for the restructuring of these NPLs.
Currently, call center efforts are largely reactive. Someone calls in, they are upset and humans respond accordingly, not always in the most effective way. Algorithms can be used to rapidly evaluate and identify the behavioral patterns of these customers – based on previous calls and observed emotional data during a call. This enables faster call pairing to the best-suited customer service agent to address the specific problem.
One can also create predictive models for individual customers based on already established demographics like their age, the type of work they are in (and the likely impact of the current crisis on that line of work), their job title and salary, and a detailed history of their recent interactions with the bank. This allows for a predictive approach to revenue recovery – identifying high-risk debtors for restructuring and deprioritizing those who are considered lower risk.
Emotion AI and AI-Mediated Conversations Redefine Bank’s Revenues Through its Call Center
Behavioral Signals has worked with several banks to address their revenue recovery and customer analysis, including those that were particularly hard hit after the 2009 financial crisis.
One of our clients, a Bank in the EU (name withheld for confidentiality) had to take measures to restructure the non-performing loans under a parliament-approved securitization scheme. The Bank uses its in-house call center to contact non-performing loan debtors and negotiate offers to restructure the debt according to the debtor’s unique situation and their ability to pay-out the loan.
Given the effect of non-performing loans (NPLs) on its bottom line, the Bank was seeking a technology partner to help it optimize the collections process. Behavioral Signals partnered with this Bank and applied its AI-based agent-customer matching technology towards maximizing the effectiveness of their Call Center.
Behavioral Signals proposed a model to optimize the call centers’ outcomes by using machine learning techniques to route the caller to the best-matching agent. By creating agent and customer behavioral profiles, from their past audio interactions, we were able to create a predictive model on which agents’ skills and overall behavioral traits during the calls are best suited for the specific customer in order to achieve the desired result.
In several of its bank related deployments, its Emotion AI technology has achieved between 12-17% increase in revenue improvement, over 8% rise in call success ratio, and close to 10% in customer satisfaction. In one specific EU bank the result translated into an additional $7.5 Million USD, in 4 months, when compared to doing business without AI assistance. The annual upside for the Bank was projected to be $1.5M for each contact center agent or $300M in total annual restructured debt.
The Bank is extending the scope of cooperation with Behavioral Signals to deploy the AI-Mediated Conversations (agent-customer matching) application to the entire revenue recovery team along with other applications, to improve the efficiency and performance of its call center.
The Future of Banking is Ready for Implementation
One of the major concerns banks have with implementing a heavily AI-driven system, in its call centers, are data privacy, security and customer satisfaction. Security is usually guaranteed with on-premises deployment and ] through the company’s GDPR and SOC certifications. Meanwhile, the banks that deployed AI-MC, noted that these AI-assisted interactions had higher than average customer satisfaction scores as rated by the Bank’s QA team, and helped to greatly improve customer service agent performance rates.
Leveraging technology may be one of the most effective ways for banks currently looking at high volumes of debt held in order to stay ahead of the crisis. Through smarter analytics, improved customer interactions, and a predictive model to identify those who need intervention sooner, banks can be better prepared for the fallout we’re about to face.
#revenuerecovery #revenuerecovery #finance #debtmanagement #covid19 #NPLs
First publication: 5/14/2020
Updated: 2/2/21