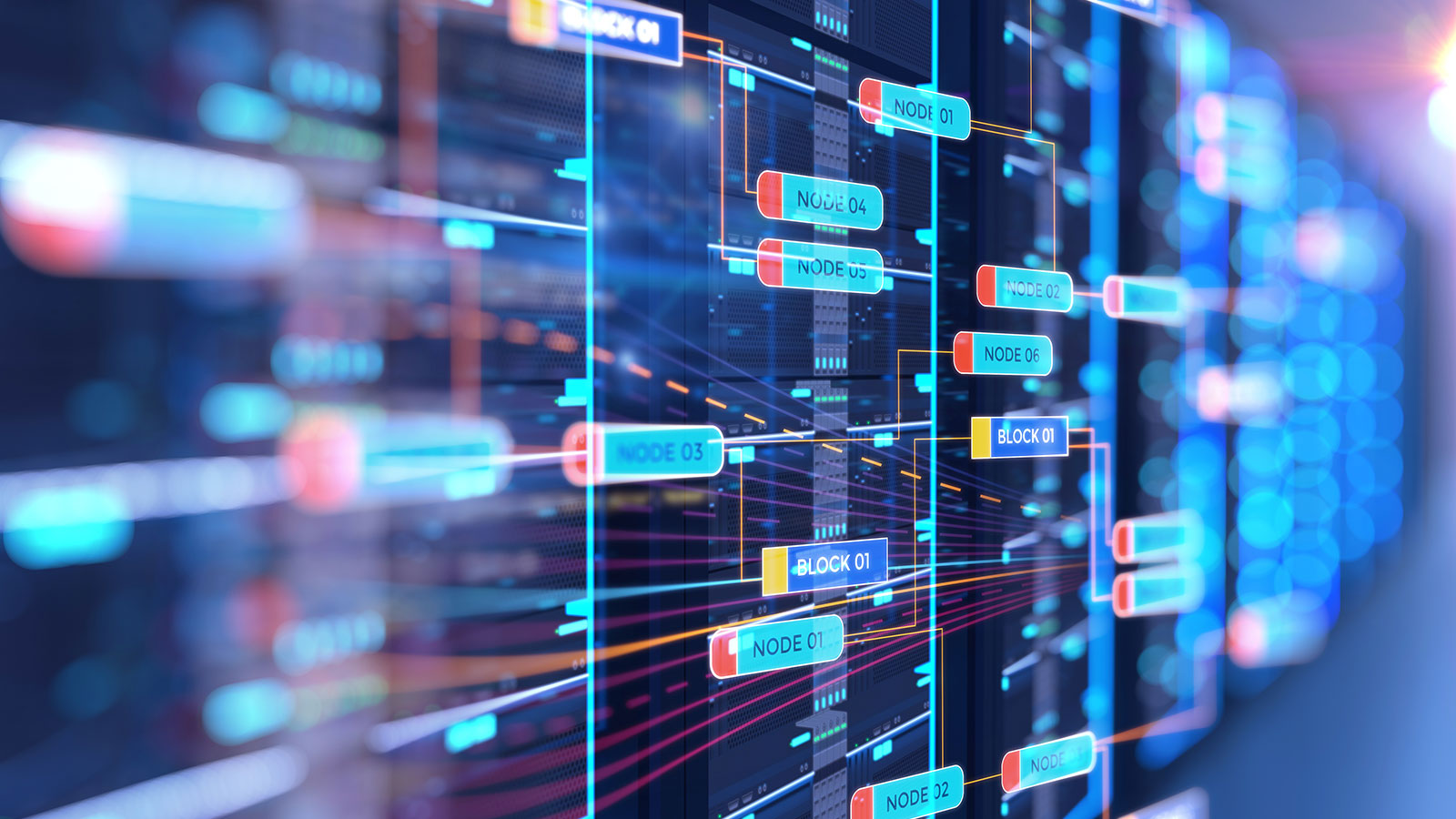
Collecting Data Alone… Is Not Enough
Technology has given us a path to solving the world’s toughest problems. The sheer volume of data collected by the myriad devices we use every day is unfathomable. More than 2.5 quintillion bytes of data are created every day, exponentially increasing the wealth of human knowledge and understanding.
But just having the data isn’t enough. Being data-driven isn’t about crunching numbers and following the trend lines. Especially when building a new product, undertaking a major project, or revising a service offering, data doesn’t always have the answers. Instead, it is a reference marker – a tool that can guide us toward the light when all else is unclear.
Data can be the tool you use to latch on to good ideas, but it should never be the sole arbiter of what is a good idea. Companies fall into this trap repeatedly, letting the data guide them in directions that might run counter to their original mission and values. Instead of being blindly led, they need to use it as one of many subjective and objective tools to improve performance.
The Key to Success Is Understanding Customers
More than ever before, we are able to capture and analyze data about the customer journey. Chatbots, AI-agent assistants and other AI-powered tools provide actionable insights in real-time for the customer service and product development teams. While conversational AI is growing more sophisticated and can provide key insights, there is still very much a human element to understanding how something makes a customer feel – whether positive or negative – and acting on that knowledge subjectively. There isn’t always a clear yes or no answer hidden in the data.
Artificial intelligence is attempting to fill this gap, however. Already, companies like Allstate, IBM, and MetLife have implemented tools that monitor and respond to cues in their customers’ speech, responding in kind by providing actionable insights to customer service agents. Behavioral Signals has been building algorithms that evaluate vocal cues that can be leveraged in a variety of applications, from customer support to research for new product development. The bottom line, though, is that without that fundamental understanding of customers, data is just noise. It can drive us in the wrong direction if not harnessed effectively.
Providing a Better Benefits Package to Employees
Another area in which raw data is plentiful but insights continue to struggle is HR. Retention remains one of the most significant challenges faced by companies of almost any size, and in some industries, the war to acquire top tier talent is only heating up. Benefits are one of the primary ways that employers entice the best employees to sign on and stay with the company, but historically, it has been something of a guessing game as to what benefits are most relevant to certain employees.
AI is changing that. With an AI-empowered system and a digitally delivered benefits-package, employers can now analyze factors like personal interests, demographics, location, health history, and major life events to prepare benefits packages specifically catered to individual employees and their families. Rather than offering blanket packages, or spending hours personally building them from scratch, all the while still guessing at what will really resonate with an employee, AI is providing those insights.
At the same time, HR specialists who currently spend 29% of their time on paperwork and administration could open up their schedules to invest in greater efforts in relationship-building and workplace wellness programs that similarly increase retention and make a company a more interesting and exciting place to work.
Gathering the Right Insights for the Right Times
For a product to be successful, its developers need to fully understand the customer, their experience and their expectations. Data can actually get in the way of this, generating excess noise that amplifies minor issues above more complex, nuanced issues that have a greater impact on adoption and retention.
For example, fitness tracking apps tend to be used more regularly by people who exercise with others. It doesn’t necessarily mean other people are less active. It just means they are less likely to remember to turn on their tracker when they go for a run or show off their performance through an app’s built-in social media integration. There’s a definitive social element to exercise – people want to share their progress, both to show what they can do and to keep themselves accountable. This “human” element is present in dozens of applications, but it isn’t always immediately available in the data.
This is where AI comes in. Through machine learning, we are increasingly able to train systems to review large data sets and identify common correlations that impact user patterns and trends. Does the time of the year impact how often someone uses a certain financial app? Are people more likely to upload photos in the evening before they go out or after a long night out with friends? These are the types of questions that traditional analysis often struggles to answer, if only because it requires someone to ask the question. With AI, the questions don’t need to be asked.
The Future of AI is Insightful
Whether evaluating voice cues and common responses from customers on service calls, preparing bespoke benefits packages based on a deep-dive analysis of employee data, or better understanding when and how customers use a product, AI is providing a new and more flexible way to analyze extremely large data sets.